Basic usage of activity is: Creation/setup of view which will used for drawing; setup of UI interaction (On Touch listener,etc.); magnetometer sensor setup; initialization of various classes used for project (such as Storage of app data, MenuManager for showing user menu, etc.); starting some threads for program state monitoring.
Used for saving information about current active menu item in UI, such as - menu text and program state which will be fired when user will switch to this menu item. (All communication between application components goes through ProgramState object, it's like a state machine :-) )
Container for MenuItem class instances. Also holds logic for controlling menu system such as - switching current active menu item, loading menu bitmap resources, checking mouse click event and performing menu actions (changing active menu item, executing current item, etc.).
Holds class MovementStatistics, which is used for computing some information about current movement path, such as - time traveled in some compass direction, times user rotated clockwise or counter-clockwise, etc. Movement class itself stores LinkedList of user moves, appends nodes to this list as user travels in some direction, communicates to magnetometer sensor fetching current user direction relative to North, converts movement data into representation suitable for drawing movement path in a view (returns Path draw object for canvas).
Implements SensorEventListener interface for getting data from magnetometer sensor. Performs all interaction with magnetometer sensor, such as - performing magnetometer calibration (detects accuracy of magnetometer, min/max values of magnetic field, checks for errors in calibration, for example there should be zero or minimum device tilting when in calibration mode, because tilting changes magnetometer output rapidly), calculates degrees to North pole according magnetometer field strength data and passes this information for compass drawing to view.
State machine of application - integrates all application components, so that all components could interact between each other and user interface. Also some threads monitors program state and changes it by calling "check..." method in ProgramState class (for example,- calibration monitor thread informs ProgramState when calibration is finished and UI can show menu for a user...).
Exports drawing to bitmap. Performs drawing of visual elements in view such as,- compass, status bar, menu, user movement statistics, user movement path.
Exports user path drawing to JPG file. Loads/Saves magnetometer calibration data.
Wrapper for vector algebra (for example,- calculation of dot product of vectors, angle between vectors, vector normalization, vector addition, vector rotation, etc...).
Most important classes are Orientation.java and Movement.java.
--------------------------------------------
Orientation.java
--------------------------------------------
Encapsulates control of magnetometer sensor. Now I would like to talk a bit about how direction to North is calculated. Most important fact is that absolute maximum value of magnetic field strength along X axis points to west, and absolute maximum value of magnetic field strength along Y axis points to north. I.e. X axis in magnetometer measures magnetic field strength along west-east axis and Y magnetometer axis measures magnetic field strength along north-south axis. So by having these facts we can calculate direction to North. Consider this picture:
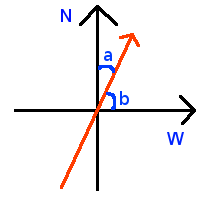
So by having magnetometer data we can calculate (a,b) angles in a following way:
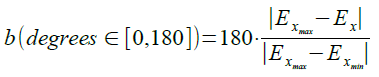
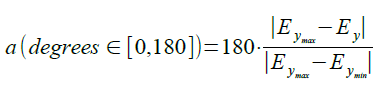
The only problem is that calculated angle a is in range 0,180 degrees, and we need full range of -180,+180 degrees. So we need to decide sign of a angle, which can be determined from angle b. By doing that we get full direction to North angle formula:

So we just need to find min,max values of magnetic field. This is achieved by magnetometer calibration in two phases. Phase 1 - simply measures fluctuation of magnetic field when device is stationary and extracts error of magnetic field magnitude. And second phase of calibration - collects magnetic field strength data when user is turning around itself (i.e. around Z axis). After 1 full rotation min,max values are extracted from this data.
-----------------------------------------
Movement.java
-----------------------------------------
This class collects user movement information in a form - How much time user traveled in some direction relative to North, by fetching magnetometer data using Orientation.java class. Later view which draws user movement path queries draw data from Movement.java class by calling method "getMovementDataForDraw()", which looks like this:
public Object[] getMovementDataForDraw(float[] startPoint) { if (moves == null || moves.size() < 2) return null; if (this.isMovementStatisticsNeeded) this.movementStats.calculateMovementStatistics(moves); LinkedListI think that this peace of code is self-descriptive, so I will not make many comments on this :-)points = convertMovesToPoints(startPoint); float[] limits = getMinMaxCoordsOfPoints(points); centerPathToScreen(points, startPoint, limits); LinkedList pointsFiltered = filterOutLinesWithSmallAngleChange(points); Path path = convertPointsToPath(pointsFiltered); Object[] ret = new Object[3]; ret[0] = path; ret[1] = pointsFiltered.getLast(); ret[2] = this.movementStats; return ret; }
And finally - some picture describing How this movement path looks like when application runs on Android-enabled device. Using this app I walked near the walls of my flat, so by doing this in the end we get my flat scheme, which looks like this:
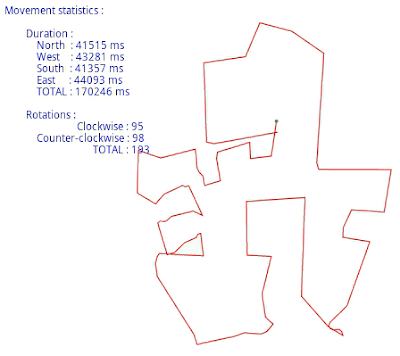
Some explanations,- starting from lower-right corner and going clockwise in picture you will get such rooms: kitchen, bedroom, WC, bathroom, sitting-room.
Have fun developing for Android and using sensors !